Learning from the Exploratories to make predictions beyond them
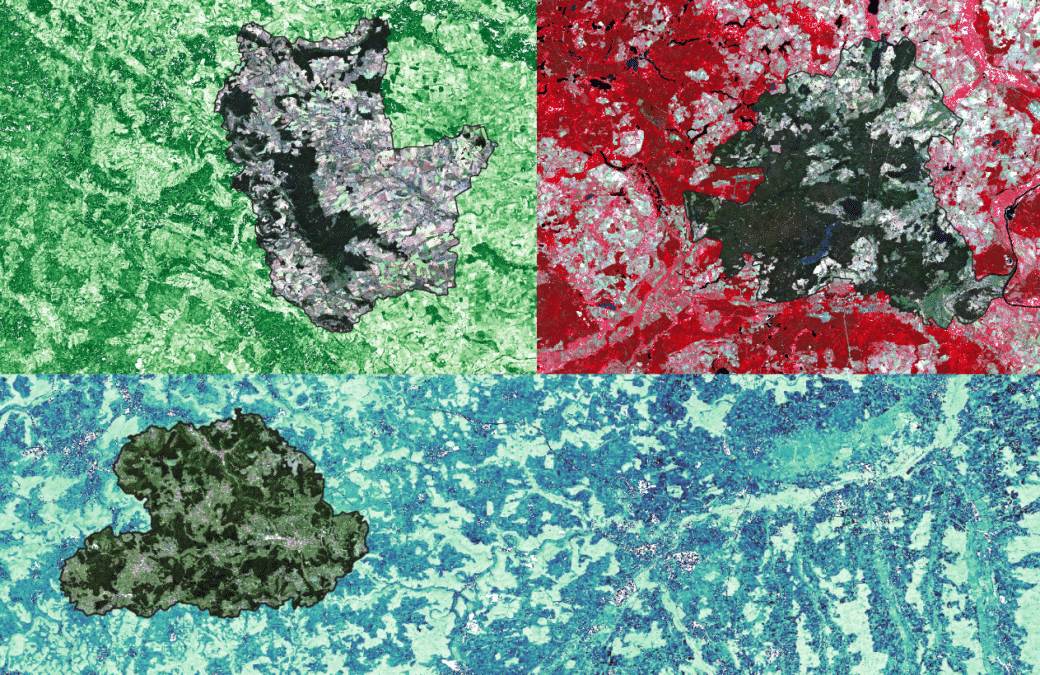
The prediction, understanding and monitoring of spatio-temporal biodiversity and ecosystem functioning patterns at the landscape-scale is a major challenge in ecological research, both for scientific objectives as well as for planning and land management. The relevance of this issue is even increasing in the context of the ongoing climate and biodiversity crisis. The many years of research in the Biodiversity Exploratories provide an unprecedented comprehensive set of point data that can be used as an ideal baseline to study biodiversity patterns in space and time at a landscape scale, and which might possibly even allow for predictions beyond the core study areas. To use the Exploratories to predict and understand biodiversity patterns beyond the core study areas, upscaling approaches are required that use field samples from the experimental plots as a baseline to gain landscape-scale and temporally continuous information. Machine learning offers great opportunities for predictive mapping, due to the ability to learn non-linear and complex relationships between drivers and biodiversity variables. However, recent research indicates considerable challenges when trained models are applied to make predictions beyond intensively studied areas and that will be addressed within the project BEyond.
With a focus on grasslands, we aim at learning from the Biodiversity Exploratories to make spatio-temporal predictions of biodiversity and ecosystem functioning variables beyond the experimental plots and beyond the core study areas of the Exploratories. However, we argue that the value of predictions is limited without knowledge on why specific predictions were made. Here, we explicitly aim at going beyond predictions and explore what models have learned and why local predictions were made, with the aim to contribute to a more mechanistic understanding of biodiversity and ecosystem functioning patterns at landscape scale.
We choose an indirect modelling approach by including terrain, soil, climate, landuse and landscape structure as potential drivers in addition to remotely sensed data. Machine learning offers great opportunities for predictive mapping, due to the ability to learn non-linear and complex relationships between drivers and biodiversity variables. However, spatial overfitting and the learning of scientifically wrong relationships may significantly lead to a limited model transferability. A lack of model interpretability further prevents advancements for ecological research. To overcome these limitations we will develop and apply new methods for spatio-temporal predictive mapping that focus on increasing the model transferability coupled with methods of explainable artificial intelligence. We expect to be able to develop scientifically sound models that provide spatio-temporal continuous maps of selected biodiversity variables beyond the Exploratories. We further go beyond predictions and expect that insights into the former “black-box” models provide novel findings on patterns, drivers and interactions. We will validate our predictions by a “rapid survey” where 150 plots are randomly chosen from the entire landscape unit around the Exploratories.